Uncovering the Potential: AI-Driven Predictive Analytics in Engineering Projects
- Neo IT Staffing Staff
- Mar 6
- 5 min read
In today's fast-paced world, data is everywhere, and technology is advancing like never before. Engineering professionals are increasingly adopting new tools to enhance project outcomes. Among these tools, AI-driven predictive analytics stands out as a game changer. This technology reshapes how engineers approach design, development, and project management by turning raw data into powerful insights.
This blog post explores the various dimensions of AI-powered predictive analytics, its implications for engineering projects, and how it can improve decision-making processes, streamline operations, and ultimately drive success.
The Foundation of AI-Driven Predictive Analytics
AI-driven predictive analytics leverages artificial intelligence algorithms and machine learning models to sift through historical data, identify patterns, and generate forecasts about future outcomes. In engineering, this translates into using enormous amounts of data gathered throughout a project’s lifecycle to predict potential challenges, risks, and opportunities.
Given that modern engineering projects are more complex than ever, traditional planning and implementation methods can fall short. Predictive analytics enhances human decision-making by providing a proactive approach based on data analysis.
The Role of Data in Predictive Analytics
The power of predictive analytics hinges on the quality and variety of available data. Engineering projects produce a diverse array of data types, such as:
Design specifications
Construction schedules
Resource allocation details
Equipment performance reports
Market conditions
By aggregating data from these different sources, AI models can uncover insights that might otherwise remain hidden. For instance, a construction project that tracks weather, equipment status, and personnel availability can significantly reduce delays—the use of such comprehensive data sources can improve project completion rates by up to 20%.
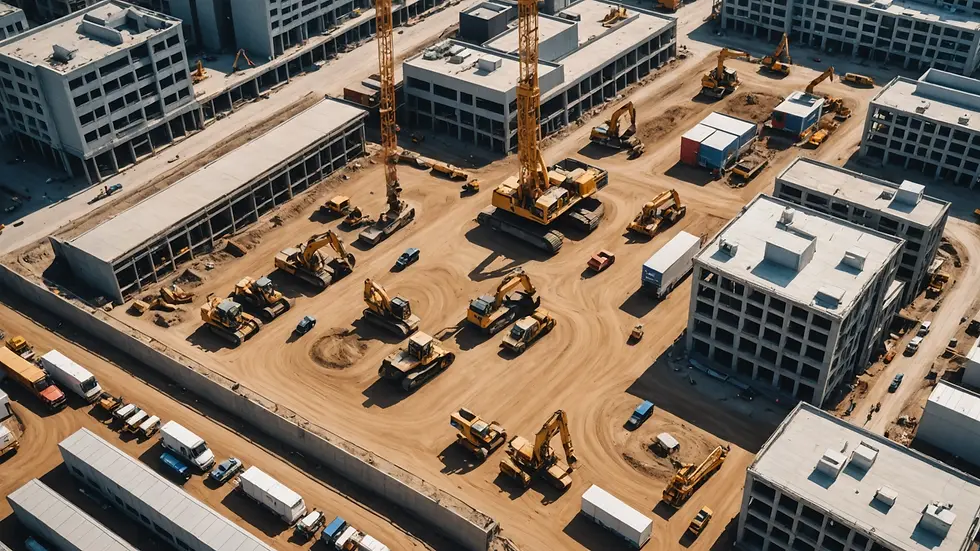
Key Benefits of AI-Driven Predictive Analytics in Engineering
The integration of AI-driven predictive analytics offers numerous advantages that can profoundly affect engineering projects. Notable benefits include:
1. Improved Risk Management
Risk management stands at the core of every successful engineering project. Predictive analytics enhances risk evaluation accuracy by identifying past project issues and estimating their likelihood of happening again. For instance, a construction firm that analyzes data from previous projects can preemptively address issues that caused delays in 30% of past initiatives. Continuous monitoring through AI allows project managers to receive alerts about potential risks in real time, enabling them to implement mitigation strategies promptly, which can decrease delays by approximately 15%.
2. Enhanced Project Planning and Scheduling
Creating effective timelines that account for possible delays can be challenging in engineering projects. Predictive analytics helps optimize project schedules by analyzing factors like resource availability and weather conditions. For example, by utilizing historical weather data, predictive models can forecast unfavorable weather, allowing managers to make schedule adjustments. Companies that have adopted these methods have reported improvements in adherence to project timelines by 25%.
3. Resource Optimization
Efficient resource allocation is critical in engineering, where every dollar counts. Predictive analytics enables organizations to determine the optimal use of resources, whether human, equipment, or materials. By reviewing historical data, AI can suggest resource distribution methods that minimize waste and boost productivity. This approach could lead to a 30% cut in unnecessary costs while ensuring timely project delivery.
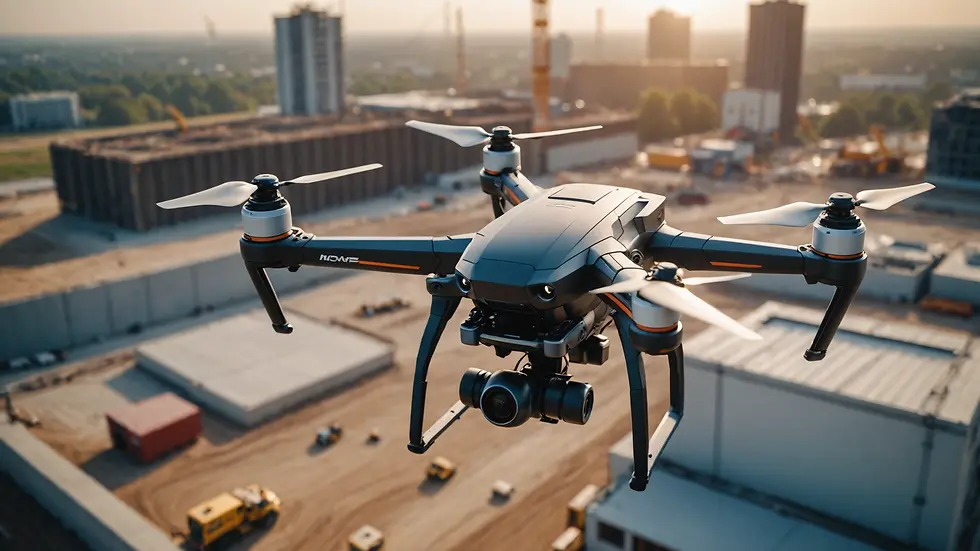
4. Quality Assurance
Maintaining quality standards is an ongoing challenge in engineering. AI-driven predictive analytics can efficiently monitor performance metrics, identifying potential quality issues before they escalate. By continuously analyzing data, AI can flag deviations from quality norms. This proactive approach can lead to a reduction in rework costs by up to 20%, ensuring projects consistently meet the desired quality outcomes.
5. Faster Decision-Making
In an industry where timing is everything, quick decision-making is vital to prevent costly delays. Predictive analytics accelerates this process by providing relevant insights at a moment’s notice. Engineers can swiftly assess various scenarios based on data-driven forecasts, boosting their capacity to make informed decisions. Streamlined operations can improve project delivery speed by 15%.
Real-World Applications of AI-Driven Predictive Analytics
Various engineering sectors have begun leveraging AI-driven predictive analytics to enhance project outcomes. Here are some key applications:
1. Civil Engineering
In civil engineering, predictive analytics is crucial for assessing traffic patterns, structural integrity, and material performance. For example, predictive models can help forecast maintenance needs for bridges and roads by analyzing factors like wear and tear over time. By identifying potential issues early, engineers can prolong infrastructure life cycles, which can enhance public safety and reduce costs.
2. Manufacturing and Construction
The manufacturing sector utilizes predictive analytics to streamline production schedules and supply chain management. By tapping into historical data, factories can predict equipment failures and schedule maintenance to minimize downtime. For instance, predictive analytics can help a factory reduce unexpected downtimes by 25% by anticipating equipment needs.
In construction, this technology allows for accurate forecasting of labor and material requirements by analyzing different project scopes, significantly reducing risk of delays and excessive expenditures.
3. Software Engineering
In software development, predictive analytics can enhance the testing phase by identifying potential defects and performance pitfalls. Analyzing historical bug data allows AI models to highlight vulnerable areas in the code, enabling developers to focus their efforts effectively. This targeted approach can decrease the time spent on testing by up to 30% while improving software quality.

Implementing AI-Driven Predictive Analytics in Engineering Projects
While AI-driven predictive analytics offers impressive benefits, implementing this technology requires thorough planning. Here’s how organizations can effectively adopt predictive analytics:
1. Assess Data Quality and Availability
Organizations should begin by evaluating their data quality and accessibility. High-quality and clean data is necessary for effective analytics. This might involve improving data collection processes or investing in better data management systems.
2. Choose the Right Tools and Technologies
Selecting user-friendly and scalable AI tools is vital for successful analytics implementation. Organizations should seek solutions that integrate seamlessly with their existing systems and offer solid analytical capabilities.
3. Train the Team
Investing in training is crucial for success. Engineering teams need to understand data analytics principles and the specific technologies being utilized. Ensuring that staff has the necessary knowledge to leverage predictive analytics can significantly enhance organizational capabilities.
4. Start Small and Scale
Organizations should consider piloting small-scale projects that allow them to explore predictive analytics without becoming overwhelmed. Once the team gains experience and confidence, they can scale up to larger engineering projects.
5. Monitor and Continuous Improvement
Implementation is an ongoing process. Continuous monitoring of predictive tools and performance is essential. Organizations should set metrics to evaluate success and consistently seek ways to refine predictive models and processes.
Challenges and Considerations
While AI-driven predictive analytics presents significant opportunities, several challenges remain when integrating these tools into engineering projects. Key considerations include:
1. Data Privacy and Security
As dependence on large datasets grows, so do concerns about data privacy and security. Organizations must put protocols in place to protect sensitive information and adhere to regulations.
2. Resistance to Change
Adopting new technologies can meet resistance from team members. Effective communication about the benefits of predictive analytics and involving employees in the process can help alleviate concerns about change.
3. Skill Gaps
A lack of skilled personnel who can interpret and act upon predictive analytics results may hinder adoption. Investing in training programs is essential to bridge this gap and enable effective use of AI tools.
Harnessing the Power of Predictive Analytics
AI-driven predictive analytics is revolutionizing the engineering sector by improving decision-making and risk management. Embracing data-driven strategies is becoming essential for success as the engineering landscape evolves.
Organizations integrating predictive analytics gain a competitive edge, optimizing resources and enhancing project outcomes. By recognizing challenges and investing in the right tools, training, and processes, professionals can leverage this transformative technology.
In a world where decisions are increasingly driven by data, utilizing AI-driven predictive analytics is not just beneficial—it is becoming essential for achieving engineering excellence. Embrace the future and let data shape your engineering projects for remarkable results.
Comentários